结合 Comet 使用 Tune#
Comet 是一个管理和优化整个 ML(机器学习)生命周期的工具,涵盖从实验跟踪、模型优化和数据集版本控制到模型生产监控等各个方面。
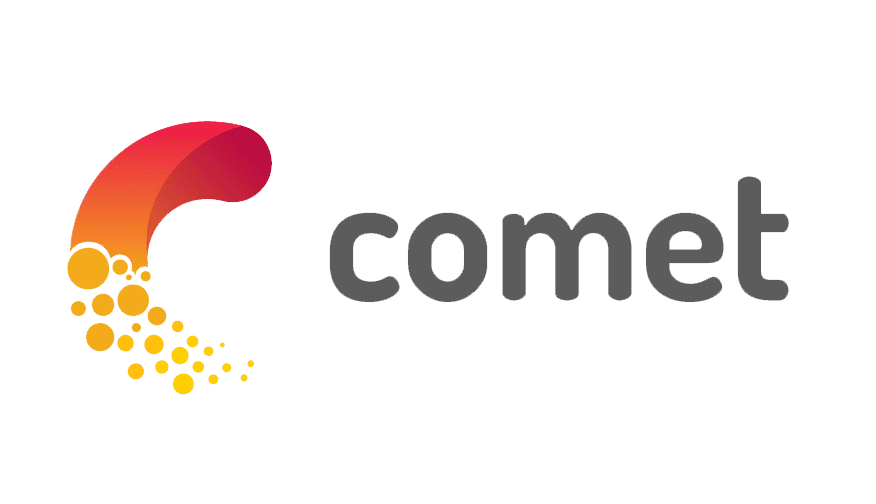
示例#
为了说明如何将 Trial 结果记录到 Comet,我们将定义一个模拟 loss
指标的简单训练函数
import numpy as np
from ray import tune
def train_function(config):
for i in range(30):
loss = config["mean"] + config["sd"] * np.random.randn()
tune.report({"loss": loss})
现在,假设您提供了 Comet API 密钥和项目名称,如下所示
api_key = "YOUR_COMET_API_KEY"
project_name = "YOUR_COMET_PROJECT_NAME"
您可以通过相应地在 RunConfig()
中指定 callbacks
参数来添加 Comet 日志记录器
from ray.air.integrations.comet import CometLoggerCallback
tuner = tune.Tuner(
train_function,
tune_config=tune.TuneConfig(
metric="loss",
mode="min",
),
run_config=tune.RunConfig(
callbacks=[
CometLoggerCallback(
api_key=api_key, project_name=project_name, tags=["comet_example"]
)
],
),
param_space={"mean": tune.grid_search([1, 2, 3]), "sd": tune.uniform(0.2, 0.8)},
)
results = tuner.fit()
print(results.get_best_result().config)
2022-07-22 15:41:21,477 INFO services.py:1483 -- View the Ray dashboard at http://127.0.0.1:8267
/Users/kai/coding/ray/python/ray/tune/trainable/function_trainable.py:643: DeprecationWarning: `checkpoint_dir` in `func(config, checkpoint_dir)` is being deprecated. To save and load checkpoint in trainable functions, please use the `ray.air.session` API:
from ray.air import session
def train(config):
# ...
session.report({"metric": metric}, checkpoint=checkpoint)
For more information please see https://docs.rayai.org.cn/en/master/ray-air/key-concepts.html#session
DeprecationWarning,
当前时间:2022-07-22 15:41:31 (已运行 00:00:06.73)
此节点内存使用量:9.9/16.0 GiB
正在使用 FIFO 调度算法。
请求资源:0/16 CPU,0/0 GPU,0.0/4.5 GiB 堆内存,0.0/2.0 GiB 对象
当前最佳 Trial:5bf98_00000,损失为 1.0234101880766688,参数为={'mean': 1, 'sd': 0.40575843135279466}
结果日志目录:/Users/kai/ray_results/train_function_2022-07-22_15-41-18
Trial 数量:3/3 (3 已终止)
Trial 名称 | 状态 | 位置 | 均值 | 标准差 | 迭代次数 | 总时间 (秒) | 损失 |
---|---|---|---|---|---|---|---|
train_function_5bf98_00000 | 已终止 | 127.0.0.1:48140 | 1 | 0.405758 | 30 | 2.11758 | 1.02341 |
train_function_5bf98_00001 | 已终止 | 127.0.0.1:48147 | 2 | 0.647335 | 30 | 0.0770731 | 1.53993 |
train_function_5bf98_00002 | 已终止 | 127.0.0.1:48151 | 3 | 0.256568 | 30 | 0.0728431 | 3.0393 |
2022-07-22 15:41:24,693 INFO plugin_schema_manager.py:52 -- Loading the default runtime env schemas: ['/Users/kai/coding/ray/python/ray/_private/runtime_env/../../runtime_env/schemas/working_dir_schema.json', '/Users/kai/coding/ray/python/ray/_private/runtime_env/../../runtime_env/schemas/pip_schema.json'].
COMET WARNING: As you are running in a Jupyter environment, you will need to call `experiment.end()` when finished to ensure all metrics and code are logged before exiting.
COMET ERROR: The given API key abc is invalid, please check it against the dashboard. Your experiment would not be logged
For more details, please refer to: https://www.comet.ml/docs/python-sdk/warnings-errors/
COMET WARNING: As you are running in a Jupyter environment, you will need to call `experiment.end()` when finished to ensure all metrics and code are logged before exiting.
COMET ERROR: The given API key abc is invalid, please check it against the dashboard. Your experiment would not be logged
For more details, please refer to: https://www.comet.ml/docs/python-sdk/warnings-errors/
COMET WARNING: As you are running in a Jupyter environment, you will need to call `experiment.end()` when finished to ensure all metrics and code are logged before exiting.
COMET ERROR: The given API key abc is invalid, please check it against the dashboard. Your experiment would not be logged
For more details, please refer to: https://www.comet.ml/docs/python-sdk/warnings-errors/
Result for train_function_5bf98_00000:
date: 2022-07-22_15-41-27
done: false
experiment_id: c94e6cdedd4540e4b40e4a34fbbeb850
hostname: Kais-MacBook-Pro.local
iterations_since_restore: 1
loss: 1.1009860426725162
node_ip: 127.0.0.1
pid: 48140
time_since_restore: 0.000125885009765625
time_this_iter_s: 0.000125885009765625
time_total_s: 0.000125885009765625
timestamp: 1658500887
timesteps_since_restore: 0
training_iteration: 1
trial_id: 5bf98_00000
warmup_time: 0.0029532909393310547
Result for train_function_5bf98_00000:
date: 2022-07-22_15-41-29
done: true
experiment_id: c94e6cdedd4540e4b40e4a34fbbeb850
experiment_tag: 0_mean=1,sd=0.4058
hostname: Kais-MacBook-Pro.local
iterations_since_restore: 30
loss: 1.0234101880766688
node_ip: 127.0.0.1
pid: 48140
time_since_restore: 2.1175789833068848
time_this_iter_s: 0.0022211074829101562
time_total_s: 2.1175789833068848
timestamp: 1658500889
timesteps_since_restore: 0
training_iteration: 30
trial_id: 5bf98_00000
warmup_time: 0.0029532909393310547
Result for train_function_5bf98_00001:
date: 2022-07-22_15-41-30
done: false
experiment_id: ba865bc613d94413a37fe027123ba031
hostname: Kais-MacBook-Pro.local
iterations_since_restore: 1
loss: 2.3754716847171182
node_ip: 127.0.0.1
pid: 48147
time_since_restore: 0.0001590251922607422
time_this_iter_s: 0.0001590251922607422
time_total_s: 0.0001590251922607422
timestamp: 1658500890
timesteps_since_restore: 0
training_iteration: 1
trial_id: 5bf98_00001
warmup_time: 0.0036537647247314453
Result for train_function_5bf98_00001:
date: 2022-07-22_15-41-30
done: true
experiment_id: ba865bc613d94413a37fe027123ba031
experiment_tag: 1_mean=2,sd=0.6473
hostname: Kais-MacBook-Pro.local
iterations_since_restore: 30
loss: 1.5399275480220707
node_ip: 127.0.0.1
pid: 48147
time_since_restore: 0.0770730972290039
time_this_iter_s: 0.002664804458618164
time_total_s: 0.0770730972290039
timestamp: 1658500890
timesteps_since_restore: 0
training_iteration: 30
trial_id: 5bf98_00001
warmup_time: 0.0036537647247314453
Result for train_function_5bf98_00002:
date: 2022-07-22_15-41-31
done: false
experiment_id: 2efb6f3c4d954bcab1ea4083f138008e
hostname: Kais-MacBook-Pro.local
iterations_since_restore: 1
loss: 3.204653294422825
node_ip: 127.0.0.1
pid: 48151
time_since_restore: 0.00014400482177734375
time_this_iter_s: 0.00014400482177734375
time_total_s: 0.00014400482177734375
timestamp: 1658500891
timesteps_since_restore: 0
training_iteration: 1
trial_id: 5bf98_00002
warmup_time: 0.0030150413513183594
Result for train_function_5bf98_00002:
date: 2022-07-22_15-41-31
done: true
experiment_id: 2efb6f3c4d954bcab1ea4083f138008e
experiment_tag: 2_mean=3,sd=0.2566
hostname: Kais-MacBook-Pro.local
iterations_since_restore: 30
loss: 3.0393011150182865
node_ip: 127.0.0.1
pid: 48151
time_since_restore: 0.07284307479858398
time_this_iter_s: 0.0020139217376708984
time_total_s: 0.07284307479858398
timestamp: 1658500891
timesteps_since_restore: 0
training_iteration: 30
trial_id: 5bf98_00002
warmup_time: 0.0030150413513183594
2022-07-22 15:41:31,290 INFO tune.py:738 -- Total run time: 7.36 seconds (6.72 seconds for the tuning loop).
{'mean': 1, 'sd': 0.40575843135279466}
Tune Comet 日志记录器#
Ray Tune 通过 CometLoggerCallback
提供了与 Comet 的集成,它可以自动将报告给 Tune 的指标和参数记录到 Comet UI。
点击下面的下拉菜单查看此回调 API 的详细信息
- class ray.air.integrations.comet.CometLoggerCallback(online: bool = True, tags: List[str] = None, save_checkpoints: bool = False, **experiment_kwargs)[source]
用于将 Tune 结果记录到 Comet 的 CometLoggerCallback。
Comet (https://comet.ml/site/) 是一个管理和优化整个 ML(机器学习)生命周期的工具,涵盖从实验跟踪、模型优化和数据集版本控制到模型生产监控等各个方面。
这个 Ray Tune
LoggerCallback
会将指标和参数发送到 Comet 进行跟踪。为了使用 CometLoggerCallback,您必须首先通过
pip install comet_ml
安装 Comet然后设置以下环境变量
export COMET_API_KEY=<您的 API 密钥>
或者,您也可以将 API 密钥作为参数传递给 CometLoggerCallback 构造函数。
CometLoggerCallback(api_key=<您的 API 密钥>)
- 参数:
online – 是否使用在线 (Online) 或离线 (Offline) 实验。默认为 True。
tags – 要添加到记录的实验的标签。默认为 None。
save_checkpoints – 如果为
True
,模型检查点将作为工件保存到 Comet ML。默认为False
。**experiment_kwargs – 其他关键字参数将传递给 comet_ml.Experiment (如果 online=False 则为 OfflineExperiment) 的构造函数。
有关 Experiment 和 OfflineExperiment 类的更多信息,请查阅 Comet ML 文档:https://comet.ml/site/
示例
from ray.air.integrations.comet import CometLoggerCallback tune.run( train, config=config callbacks=[CometLoggerCallback( True, ['tag1', 'tag2'], workspace='my_workspace', project_name='my_project_name' )] )