简单的并行模型选择#
提示
对于生产级别的分布式超参数调优实现,请使用 Ray Tune,一个使用 Ray Actor API 构建的可伸缩超参数调优库。
在本示例中,我们将演示如何快速编写一个并行评估一组超参数的超参数调优脚本。
该脚本将演示如何使用 Ray API 的两个重要部分:使用 ray.remote
定义远程函数,以及使用 ray.wait
等待结果就绪。
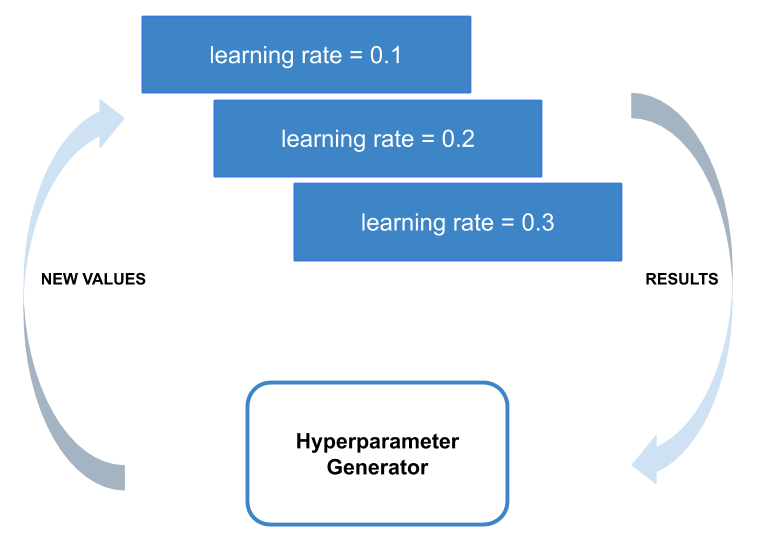
设置:依赖项#
首先,导入一些依赖项并定义用于生成随机超参数和检索数据的函数。
import os
import numpy as np
from filelock import FileLock
import torch
import torch.nn as nn
import torch.nn.functional as F
import torch.optim as optim
from torchvision import datasets, transforms
import ray
ray.init()
# The number of sets of random hyperparameters to try.
num_evaluations = 10
# A function for generating random hyperparameters.
def generate_hyperparameters():
return {
"learning_rate": 10 ** np.random.uniform(-5, 1),
"batch_size": np.random.randint(1, 100),
"momentum": np.random.uniform(0, 1),
}
def get_data_loaders(batch_size):
mnist_transforms = transforms.Compose(
[transforms.ToTensor(), transforms.Normalize((0.1307,), (0.3081,))]
)
# We add FileLock here because multiple workers will want to
# download data, and this may cause overwrites since
# DataLoader is not threadsafe.
with FileLock(os.path.expanduser("~/data.lock")):
train_loader = torch.utils.data.DataLoader(
datasets.MNIST(
"~/data", train=True, download=True, transform=mnist_transforms
),
batch_size=batch_size,
shuffle=True,
)
test_loader = torch.utils.data.DataLoader(
datasets.MNIST("~/data", train=False, transform=mnist_transforms),
batch_size=batch_size,
shuffle=True,
)
return train_loader, test_loader
设置:定义神经网络#
我们定义了一个用于训练的小型神经网络。此外,我们创建了训练和测试此神经网络的方法。
class ConvNet(nn.Module):
"""Simple two layer Convolutional Neural Network."""
def __init__(self):
super(ConvNet, self).__init__()
self.conv1 = nn.Conv2d(1, 3, kernel_size=3)
self.fc = nn.Linear(192, 10)
def forward(self, x):
x = F.relu(F.max_pool2d(self.conv1(x), 3))
x = x.view(-1, 192)
x = self.fc(x)
return F.log_softmax(x, dim=1)
def train(model, optimizer, train_loader, device=torch.device("cpu")):
"""Optimize the model with one pass over the data.
Cuts off at 1024 samples to simplify training.
"""
model.train()
for batch_idx, (data, target) in enumerate(train_loader):
if batch_idx * len(data) > 1024:
return
data, target = data.to(device), target.to(device)
optimizer.zero_grad()
output = model(data)
loss = F.nll_loss(output, target)
loss.backward()
optimizer.step()
def test(model, test_loader, device=torch.device("cpu")):
"""Checks the validation accuracy of the model.
Cuts off at 512 samples for simplicity.
"""
model.eval()
correct = 0
total = 0
with torch.no_grad():
for batch_idx, (data, target) in enumerate(test_loader):
if batch_idx * len(data) > 512:
break
data, target = data.to(device), target.to(device)
outputs = model(data)
_, predicted = torch.max(outputs.data, 1)
total += target.size(0)
correct += (predicted == target).sum().item()
return correct / total
评估超参数#
对于给定的配置,先前创建的神经网络将被训练并返回模型的准确率。然后将测试这些训练过的网络的准确率,以找到最佳的超参数集合。
@ray.remote
装饰器定义了一个远程进程。
@ray.remote
def evaluate_hyperparameters(config):
model = ConvNet()
train_loader, test_loader = get_data_loaders(config["batch_size"])
optimizer = optim.SGD(
model.parameters(), lr=config["learning_rate"], momentum=config["momentum"]
)
train(model, optimizer, train_loader)
return test(model, test_loader)
随机生成超参数的同步评估#
我们将为我们的神经网络创建多组随机超参数,这些超参数将并行评估。
# Keep track of the best hyperparameters and the best accuracy.
best_hyperparameters = None
best_accuracy = 0
# A list holding the object refs for all of the experiments that we have
# launched but have not yet been processed.
remaining_ids = []
# A dictionary mapping an experiment's object ref to its hyperparameters.
# hyerparameters used for that experiment.
hyperparameters_mapping = {}
启动用于评估不同超参数的异步并行任务。accuracy_id
是一个 ObjectRef,它充当远程任务的句柄。稍后在任务完成后使用它来获取任务结果。
# Randomly generate sets of hyperparameters and launch a task to evaluate it.
for i in range(num_evaluations):
hyperparameters = generate_hyperparameters()
accuracy_id = evaluate_hyperparameters.remote(hyperparameters)
remaining_ids.append(accuracy_id)
hyperparameters_mapping[accuracy_id] = hyperparameters
按超参数和相应准确率完成的顺序处理它们,以存储具有最佳准确率的超参数。
# Fetch and print the results of the tasks in the order that they complete.
while remaining_ids:
# Use ray.wait to get the object ref of the first task that completes.
done_ids, remaining_ids = ray.wait(remaining_ids)
# There is only one return result by default.
result_id = done_ids[0]
hyperparameters = hyperparameters_mapping[result_id]
accuracy = ray.get(result_id)
print(
"""We achieve accuracy {:.3}% with
learning_rate: {:.2}
batch_size: {}
momentum: {:.2}
""".format(
100 * accuracy,
hyperparameters["learning_rate"],
hyperparameters["batch_size"],
hyperparameters["momentum"],
)
)
if accuracy > best_accuracy:
best_hyperparameters = hyperparameters
best_accuracy = accuracy
# Record the best performing set of hyperparameters.
print(
"""Best accuracy over {} trials was {:.3} with
learning_rate: {:.2}
batch_size: {}
momentum: {:.2}
""".format(
num_evaluations,
100 * best_accuracy,
best_hyperparameters["learning_rate"],
best_hyperparameters["batch_size"],
best_hyperparameters["momentum"],
)
)