保存和加载检查点#
Ray Train 提供了使用 检查点 (Checkpoints)
记录训练进度快照的方法。
这对于以下方面很有用:
存储性能最佳的模型权重:将模型保存到持久化存储中,并用于下游服务或推理。
容错:处理长时间运行的训练作业中的工作进程和节点故障,并利用可抢占机器。
分布式检查点:Ray Train 检查点可用于从多个工作进程并行上传模型分片。
训练期间保存检查点#
一个 检查点 (Checkpoint)
是 Ray Train 提供的一个轻量级接口,它表示本地或远程存储上的一个目录。
例如,检查点可以指向云存储中的一个目录:s3://my-bucket/my-checkpoint-dir
。本地可用的检查点指向本地文件系统上的一个位置:/tmp/my-checkpoint-dir
。
以下是如何在训练循环中保存检查点:
将模型检查点写入本地目录。
由于
检查点 (Checkpoint)
仅指向一个目录,其内容完全由你决定。这意味着你可以使用任何你想要的序列化格式。
这使得使用训练框架提供的常用检查点工具变得容易,例如
torch.save
、pl.Trainer.save_checkpoint
、Accelerate 的accelerator.save_model
、Transformers 的save_pretrained
、tf.keras.Model.save
等。
使用
Checkpoint.from_directory
从目录创建检查点 (Checkpoint)
。使用
ray.train.report(metrics, checkpoint=...)
将检查点报告给 Ray Train。与检查点一同报告的指标用于跟踪性能最佳的检查点。
如果已配置,这将把检查点上传到持久化存储。请参阅配置持久化存储。
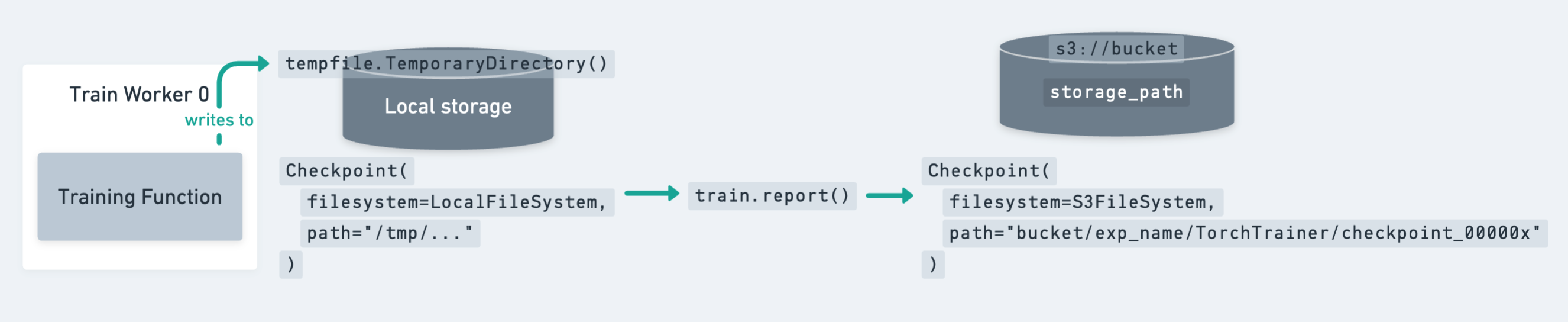
检查点 (Checkpoint)
的生命周期,从本地保存到磁盘,到通过 train.report
上传到持久化存储。#
如上图所示,保存检查点的最佳实践是先将检查点转储到本地临时目录。然后,调用 train.report
将检查点上传到其最终的持久化存储位置。之后,可以安全地清理本地临时目录以释放磁盘空间(例如,通过退出 tempfile.TemporaryDirectory
上下文)。
提示
在标准的 DDP 训练中,每个工作进程都拥有完整模型的副本,你应仅从单个工作进程保存和报告检查点,以防止冗余上传。
这通常看起来像
import tempfile
from ray import train
def train_fn(config):
...
metrics = {...}
with tempfile.TemporaryDirectory() as temp_checkpoint_dir:
checkpoint = None
# Only the global rank 0 worker saves and reports the checkpoint
if train.get_context().get_world_rank() == 0:
... # Save checkpoint to temp_checkpoint_dir
checkpoint = Checkpoint.from_directory(tmpdir)
train.report(metrics, checkpoint=checkpoint)
如果使用 DeepSpeed Zero 和 FSDP 等并行训练策略,其中每个工作进程仅拥有完整训练状态的一个分片,你可以从每个工作进程保存和报告检查点。有关示例,请参阅从多个工作进程保存检查点(分布式检查点)。
以下是使用不同训练框架保存检查点的一些示例:
import os
import tempfile
import numpy as np
import torch
import torch.nn as nn
from torch.optim import Adam
import ray.train.torch
from ray import train
from ray.train import Checkpoint, ScalingConfig
from ray.train.torch import TorchTrainer
def train_func(config):
n = 100
# create a toy dataset
# data : X - dim = (n, 4)
# target : Y - dim = (n, 1)
X = torch.Tensor(np.random.normal(0, 1, size=(n, 4)))
Y = torch.Tensor(np.random.uniform(0, 1, size=(n, 1)))
# toy neural network : 1-layer
# Wrap the model in DDP
model = ray.train.torch.prepare_model(nn.Linear(4, 1))
criterion = nn.MSELoss()
optimizer = Adam(model.parameters(), lr=3e-4)
for epoch in range(config["num_epochs"]):
y = model.forward(X)
loss = criterion(y, Y)
optimizer.zero_grad()
loss.backward()
optimizer.step()
metrics = {"loss": loss.item()}
with tempfile.TemporaryDirectory() as temp_checkpoint_dir:
checkpoint = None
should_checkpoint = epoch % config.get("checkpoint_freq", 1) == 0
# In standard DDP training, where the model is the same across all ranks,
# only the global rank 0 worker needs to save and report the checkpoint
if train.get_context().get_world_rank() == 0 and should_checkpoint:
torch.save(
model.module.state_dict(), # NOTE: Unwrap the model.
os.path.join(temp_checkpoint_dir, "model.pt"),
)
checkpoint = Checkpoint.from_directory(temp_checkpoint_dir)
train.report(metrics, checkpoint=checkpoint)
trainer = TorchTrainer(
train_func,
train_loop_config={"num_epochs": 5},
scaling_config=ScalingConfig(num_workers=2),
)
result = trainer.fit()
提示
在将 DDP 模型保存到检查点之前,你很可能希望先解包该模型。model.module.state_dict()
返回的状态字典中,每个键都没有 "module."
前缀。
Ray Train 利用 PyTorch Lightning 的 Callback
接口报告指标和检查点。我们提供了一个简单的回调实现,它在 on_train_epoch_end
时进行报告。
具体来说,在每个训练 epoch 结束时,它会:
收集来自
trainer.callback_metrics
的所有已记录指标通过
trainer.save_checkpoint
保存检查点通过
ray.train.report(metrics, checkpoint)
报告给 Ray Train
import pytorch_lightning as pl
from ray import train
from ray.train.lightning import RayTrainReportCallback
from ray.train.torch import TorchTrainer
class MyLightningModule(pl.LightningModule):
# ...
def on_validation_epoch_end(self):
...
mean_acc = calculate_accuracy()
self.log("mean_accuracy", mean_acc, sync_dist=True)
def train_func():
...
model = MyLightningModule(...)
datamodule = MyLightningDataModule(...)
trainer = pl.Trainer(
# ...
callbacks=[RayTrainReportCallback()]
)
trainer.fit(model, datamodule=datamodule)
ray_trainer = TorchTrainer(
train_func,
scaling_config=train.ScalingConfig(num_workers=2),
run_config=train.RunConfig(
checkpoint_config=train.CheckpointConfig(
num_to_keep=2,
checkpoint_score_attribute="mean_accuracy",
checkpoint_score_order="max",
),
),
)
你可以始终通过 result.checkpoint
和 result.best_checkpoints
获取已保存的检查点路径。
对于更高级的用法(例如以不同的频率报告、报告自定义检查点文件),你可以实现自己的自定义回调。这是一个简单的示例,它每 3 个 epoch 报告一次检查点:
import os
from tempfile import TemporaryDirectory
from pytorch_lightning.callbacks import Callback
import ray
import ray.train
from ray.train import Checkpoint
class CustomRayTrainReportCallback(Callback):
def on_train_epoch_end(self, trainer, pl_module):
should_checkpoint = trainer.current_epoch % 3 == 0
with TemporaryDirectory() as tmpdir:
# Fetch metrics from `self.log(..)` in the LightningModule
metrics = trainer.callback_metrics
metrics = {k: v.item() for k, v in metrics.items()}
# Add customized metrics
metrics["epoch"] = trainer.current_epoch
metrics["custom_metric"] = 123
checkpoint = None
global_rank = ray.train.get_context().get_world_rank() == 0
if global_rank == 0 and should_checkpoint:
# Save model checkpoint file to tmpdir
ckpt_path = os.path.join(tmpdir, "ckpt.pt")
trainer.save_checkpoint(ckpt_path, weights_only=False)
checkpoint = Checkpoint.from_directory(tmpdir)
# Report to train session
ray.train.report(metrics=metrics, checkpoint=checkpoint)
Ray Train 利用 HuggingFace Transformers Trainer 的 Callback
接口报告指标和检查点。
选项 1:使用 Ray Train 的默认报告回调
我们提供了一个简单的回调实现 RayTrainReportCallback
,它在检查点保存时进行报告。你可以通过 save_strategy
和 save_steps 更改检查点保存频率。它会收集最新的已记录指标,并与最新的已保存检查点一起报告。
from transformers import TrainingArguments
from ray import train
from ray.train.huggingface.transformers import RayTrainReportCallback, prepare_trainer
from ray.train.torch import TorchTrainer
def train_func(config):
...
# Configure logging, saving, evaluation strategies as usual.
args = TrainingArguments(
...,
evaluation_strategy="epoch",
save_strategy="epoch",
logging_strategy="step",
)
trainer = transformers.Trainer(args, ...)
# Add a report callback to transformers Trainer
# =============================================
trainer.add_callback(RayTrainReportCallback())
trainer = prepare_trainer(trainer)
trainer.train()
ray_trainer = TorchTrainer(
train_func,
run_config=train.RunConfig(
checkpoint_config=train.CheckpointConfig(
num_to_keep=3,
checkpoint_score_attribute="eval_loss", # The monitoring metric
checkpoint_score_order="min",
)
),
)
请注意,RayTrainReportCallback
将最新指标和检查点绑定在一起,因此用户可以正确配置 logging_strategy
、save_strategy
和 evaluation_strategy
,以确保监控指标与检查点保存步骤一致地记录。
例如,评估指标(本例中的 eval_loss
)在评估期间记录。如果用户希望根据 eval_loss
保留最好的 3 个检查点,他们应该对齐保存和评估的频率。以下是两个有效配置的示例:
args = TrainingArguments(
...,
evaluation_strategy="epoch",
save_strategy="epoch",
)
args = TrainingArguments(
...,
evaluation_strategy="steps",
save_strategy="steps",
eval_steps=50,
save_steps=100,
)
# And more ...
选项 2:实现自定义报告回调
如果你觉得 Ray Train 的默认 RayTrainReportCallback
不足以满足你的用例,你也可以自己实现一个回调!下面是一个示例实现,它收集最新指标并在检查点保存时进行报告。
from ray import train
from transformers.trainer_callback import TrainerCallback
class MyTrainReportCallback(TrainerCallback):
def __init__(self):
super().__init__()
self.metrics = {}
def on_log(self, args, state, control, model=None, logs=None, **kwargs):
"""Log is called on evaluation step and logging step."""
self.metrics.update(logs)
def on_save(self, args, state, control, **kwargs):
"""Event called after a checkpoint save."""
checkpoint = None
if train.get_context().get_world_rank() == 0:
# Build a Ray Train Checkpoint from the latest checkpoint
checkpoint_path = transformers.trainer.get_last_checkpoint(args.output_dir)
checkpoint = Checkpoint.from_directory(checkpoint_path)
# Report to Ray Train with up-to-date metrics
ray.train.report(metrics=self.metrics, checkpoint=checkpoint)
# Clear the metrics buffer
self.metrics = {}
你可以通过实现自己的 Transformers Trainer 回调来定制何时(on_save
、on_epoch_end
、on_evaluate
)以及报告什么内容(自定义指标和检查点文件)。
从多个工作进程保存检查点(分布式检查点)#
在模型并行训练策略中,每个工作进程仅拥有完整模型的一个分片,你可以从每个工作进程并行保存和报告检查点分片。
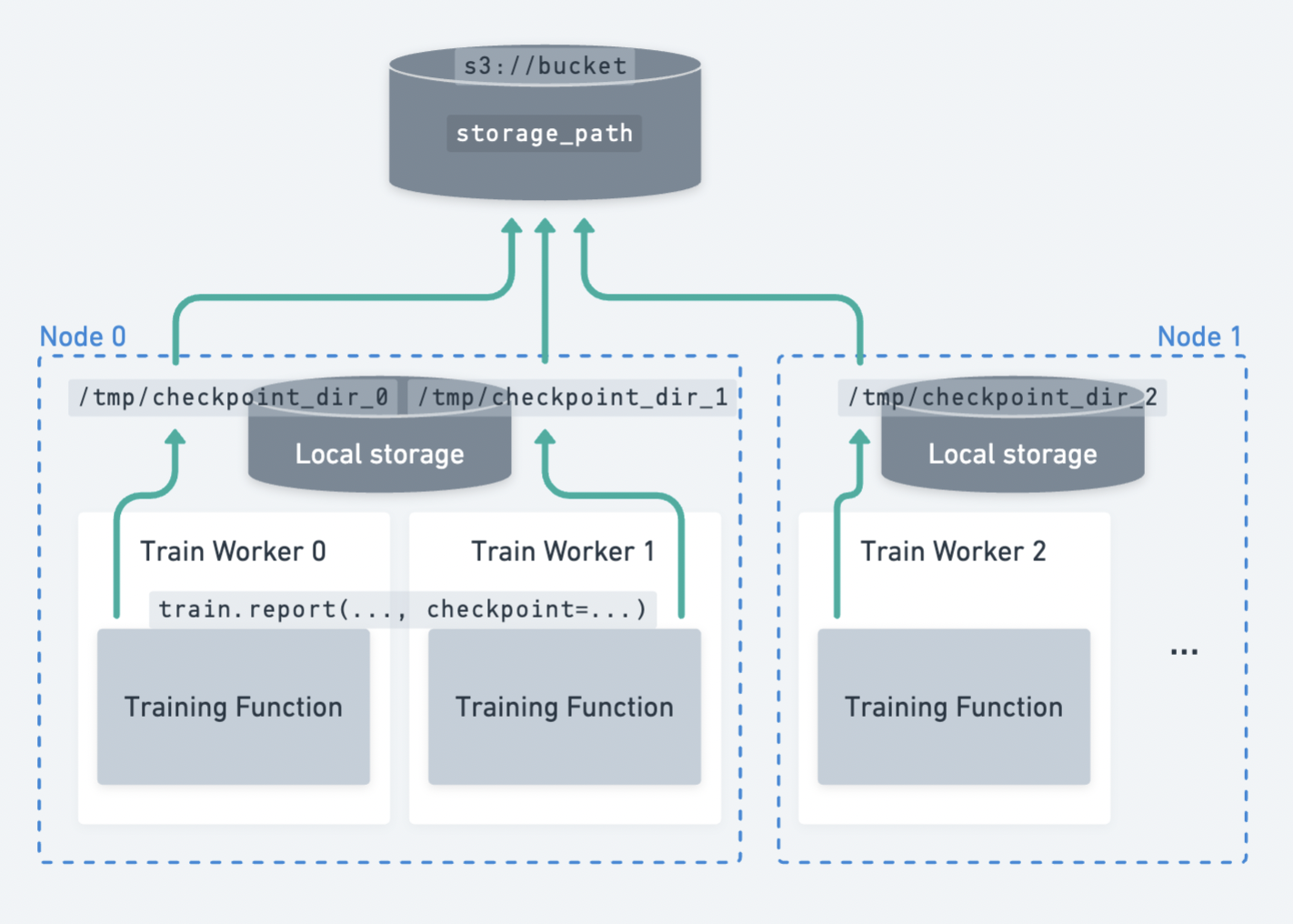
Ray Train 中的分布式检查点。每个工作进程独立地将其检查点分片上传到持久化存储。#
分布式检查点是进行模型并行训练(例如 DeepSpeed、FSDP、Megatron-LM)时保存检查点的最佳实践。
主要有两个好处:
速度更快,从而减少空闲时间。更快的检查点保存能激励更频繁地保存检查点!
每个工作进程可以并行上传其检查点分片,最大化集群的网络带宽。集群将负载分散到
N
个节点,每个节点上传大小为M / N
的分片,而不是由单个节点上传大小为M
的完整模型。分布式检查点避免了需要将完整模型聚集到单个工作进程的 CPU 内存中。
此聚集操作对执行检查点保存的工作进程提出了巨大的 CPU 内存要求,并且是 OOM 错误的常见原因。
以下是使用 PyTorch 进行分布式检查点的示例:
from ray import train
from ray.train import Checkpoint
from ray.train.torch import TorchTrainer
def train_func(config):
...
with tempfile.TemporaryDirectory() as temp_checkpoint_dir:
rank = train.get_context().get_world_rank()
torch.save(
...,
os.path.join(temp_checkpoint_dir, f"model-rank={rank}.pt"),
)
checkpoint = Checkpoint.from_directory(temp_checkpoint_dir)
train.report(metrics, checkpoint=checkpoint)
trainer = TorchTrainer(
train_func,
scaling_config=train.ScalingConfig(num_workers=2),
run_config=train.RunConfig(storage_path="s3://bucket/"),
)
# The checkpoint in cloud storage will contain: model-rank=0.pt, model-rank=1.pt
注意
同名检查点文件将在工作进程之间发生冲突。你可以通过向检查点文件添加特定于 rank 的后缀来解决此问题。
请注意,文件名冲突不会导致错误,但会导致最后上传的版本被持久化。如果所有工作进程的文件内容相同,则这没有问题。
DeepSpeed 等框架提供的模型分片保存工具已经会创建特定于 rank 的文件名,因此通常无需担心此问题。
配置检查点#
Ray Train 通过 CheckpointConfig
提供了一些检查点配置选项。主要的配置是根据某个指标仅保留前 K
个检查点。性能较差的检查点会被删除以节省存储空间。默认情况下,会保留所有检查点。
from ray.train import RunConfig, CheckpointConfig
# Example 1: Only keep the 2 *most recent* checkpoints and delete the others.
run_config = RunConfig(checkpoint_config=CheckpointConfig(num_to_keep=2))
# Example 2: Only keep the 2 *best* checkpoints and delete the others.
run_config = RunConfig(
checkpoint_config=CheckpointConfig(
num_to_keep=2,
# *Best* checkpoints are determined by these params:
checkpoint_score_attribute="mean_accuracy",
checkpoint_score_order="max",
),
# This will store checkpoints on S3.
storage_path="s3://remote-bucket/location",
)
注意
如果你想通过 CheckpointConfig
根据某个指标保存前 num_to_keep
个检查点,请确保始终将该指标与检查点一起报告。
训练后使用检查点#
可以使用 Result.checkpoint
访问最新保存的检查点。
可以使用 Result.best_checkpoints
访问所有持久化的检查点列表。如果设置了 CheckpointConfig(num_to_keep)
,此列表将包含最佳的 num_to_keep
个检查点。
有关检查训练结果的完整指南,请参阅检查训练结果。
Checkpoint.as_directory
和 Checkpoint.to_directory
是与 Train 检查点交互的两个主要 API
from pathlib import Path
from ray.train import Checkpoint
# For demonstration, create a locally available directory with a `model.pt` file.
example_checkpoint_dir = Path("/tmp/test-checkpoint")
example_checkpoint_dir.mkdir()
example_checkpoint_dir.joinpath("model.pt").touch()
# Create the checkpoint, which is a reference to the directory.
checkpoint = Checkpoint.from_directory(example_checkpoint_dir)
# Inspect the checkpoint's contents with either `as_directory` or `to_directory`:
with checkpoint.as_directory() as checkpoint_dir:
assert Path(checkpoint_dir).joinpath("model.pt").exists()
checkpoint_dir = checkpoint.to_directory()
assert Path(checkpoint_dir).joinpath("model.pt").exists()
对于 Lightning 和 Transformers,如果你在训练函数中使用默认的 RayTrainReportCallback
保存检查点,你可以如下检索原始检查点文件:
# After training finished
checkpoint = result.checkpoint
with checkpoint.as_directory() as checkpoint_dir:
lightning_checkpoint_path = f"{checkpoint_dir}/checkpoint.ckpt"
# After training finished
checkpoint = result.checkpoint
with checkpoint.as_directory() as checkpoint_dir:
hf_checkpoint_path = f"{checkpoint_dir}/checkpoint/"
从检查点恢复训练状态#
为了启用容错,你应该修改训练循环以从 检查点 (Checkpoint)
恢复训练状态。
可以在训练函数中使用 ray.train.get_checkpoint
访问用于恢复的 检查点 (Checkpoint)
。
ray.train.get_checkpoint
返回的检查点是在自动故障恢复期间填充的最新报告的检查点。
有关恢复和容错的更多详细信息,请参阅处理故障和节点抢占。
import os
import tempfile
import numpy as np
import torch
import torch.nn as nn
from torch.optim import Adam
import ray.train.torch
from ray import train
from ray.train import Checkpoint, ScalingConfig
from ray.train.torch import TorchTrainer
def train_func(config):
n = 100
# create a toy dataset
# data : X - dim = (n, 4)
# target : Y - dim = (n, 1)
X = torch.Tensor(np.random.normal(0, 1, size=(n, 4)))
Y = torch.Tensor(np.random.uniform(0, 1, size=(n, 1)))
# toy neural network : 1-layer
model = nn.Linear(4, 1)
optimizer = Adam(model.parameters(), lr=3e-4)
criterion = nn.MSELoss()
# Wrap the model in DDP and move it to GPU.
model = ray.train.torch.prepare_model(model)
# ====== Resume training state from the checkpoint. ======
start_epoch = 0
checkpoint = train.get_checkpoint()
if checkpoint:
with checkpoint.as_directory() as checkpoint_dir:
model_state_dict = torch.load(
os.path.join(checkpoint_dir, "model.pt"),
# map_location=..., # Load onto a different device if needed.
)
model.module.load_state_dict(model_state_dict)
optimizer.load_state_dict(
torch.load(os.path.join(checkpoint_dir, "optimizer.pt"))
)
start_epoch = (
torch.load(os.path.join(checkpoint_dir, "extra_state.pt"))["epoch"] + 1
)
# ========================================================
for epoch in range(start_epoch, config["num_epochs"]):
y = model.forward(X)
loss = criterion(y, Y)
optimizer.zero_grad()
loss.backward()
optimizer.step()
metrics = {"loss": loss.item()}
with tempfile.TemporaryDirectory() as temp_checkpoint_dir:
checkpoint = None
should_checkpoint = epoch % config.get("checkpoint_freq", 1) == 0
# In standard DDP training, where the model is the same across all ranks,
# only the global rank 0 worker needs to save and report the checkpoint
if train.get_context().get_world_rank() == 0 and should_checkpoint:
# === Make sure to save all state needed for resuming training ===
torch.save(
model.module.state_dict(), # NOTE: Unwrap the model.
os.path.join(temp_checkpoint_dir, "model.pt"),
)
torch.save(
optimizer.state_dict(),
os.path.join(temp_checkpoint_dir, "optimizer.pt"),
)
torch.save(
{"epoch": epoch},
os.path.join(temp_checkpoint_dir, "extra_state.pt"),
)
# ================================================================
checkpoint = Checkpoint.from_directory(temp_checkpoint_dir)
train.report(metrics, checkpoint=checkpoint)
if epoch == 1:
raise RuntimeError("Intentional error to showcase restoration!")
trainer = TorchTrainer(
train_func,
train_loop_config={"num_epochs": 5},
scaling_config=ScalingConfig(num_workers=2),
run_config=train.RunConfig(failure_config=train.FailureConfig(max_failures=1)),
)
result = trainer.fit()
import os
from ray import train
from ray.train import Checkpoint
from ray.train.torch import TorchTrainer
from ray.train.lightning import RayTrainReportCallback
def train_func():
model = MyLightningModule(...)
datamodule = MyLightningDataModule(...)
trainer = pl.Trainer(..., callbacks=[RayTrainReportCallback()])
checkpoint = train.get_checkpoint()
if checkpoint:
with checkpoint.as_directory() as ckpt_dir:
ckpt_path = os.path.join(ckpt_dir, RayTrainReportCallback.CHECKPOINT_NAME)
trainer.fit(model, datamodule=datamodule, ckpt_path=ckpt_path)
else:
trainer.fit(model, datamodule=datamodule)
ray_trainer = TorchTrainer(
train_func,
scaling_config=train.ScalingConfig(num_workers=2),
run_config=train.RunConfig(
checkpoint_config=train.CheckpointConfig(num_to_keep=2),
),
)
注意
在这些示例中,使用 Checkpoint.as_directory
将检查点内容视为本地目录。
如果检查点指向本地目录,此方法仅返回本地目录路径,不进行复制。
如果检查点指向远程目录,此方法会将检查点下载到本地临时目录,并返回临时目录的路径。
如果同一节点上的多个进程同时调用此方法,只有一个进程会执行下载,而其他进程则等待下载完成。下载完成后,所有进程都将收到相同的本地(临时)目录进行读取。
一旦所有进程都处理完检查点,临时目录将被清理。