将 LightGBM 与 Tune 一起使用#
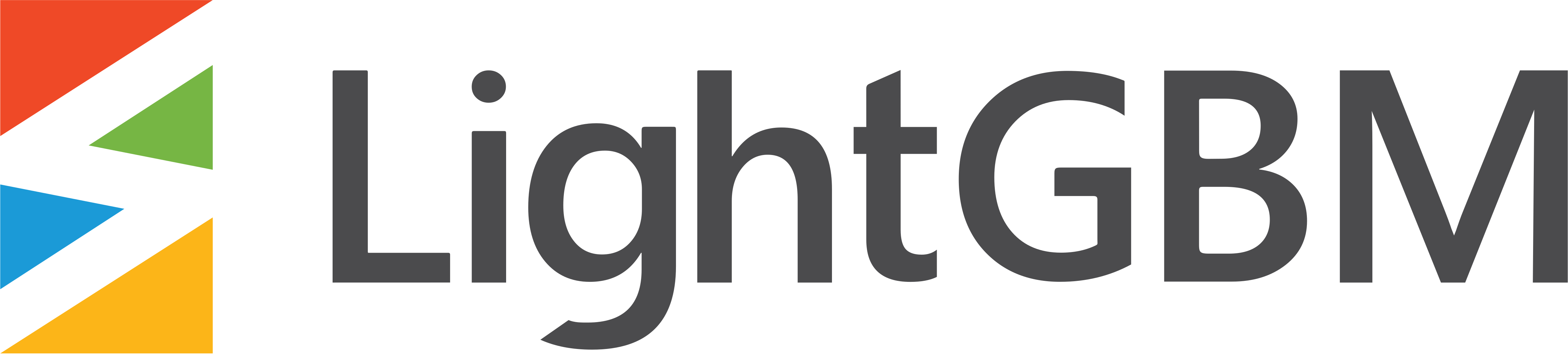
本教程展示了如何使用 Ray Tune 优化 LightGBM 模型的超参数。我们将使用 scikit-learn 中的乳腺癌分类数据集来演示如何
使用 Ray Tune 设置 LightGBM 训练函数
配置超参数搜索空间
使用 ASHA 调度器进行高效超参数调优
报告和检查点训练进度
安装#
首先,让我们安装所需的依赖项
pip install "ray[tune]" lightgbm scikit-learn numpy
import lightgbm as lgb
import numpy as np
import sklearn.datasets
import sklearn.metrics
from sklearn.model_selection import train_test_split
from ray import tune
from ray.tune.schedulers import ASHAScheduler
from ray.tune.integration.lightgbm import TuneReportCheckpointCallback
def train_breast_cancer(config):
data, target = sklearn.datasets.load_breast_cancer(return_X_y=True)
train_x, test_x, train_y, test_y = train_test_split(data, target, test_size=0.25)
train_set = lgb.Dataset(train_x, label=train_y)
test_set = lgb.Dataset(test_x, label=test_y)
gbm = lgb.train(
config,
train_set,
valid_sets=[test_set],
valid_names=["eval"],
callbacks=[
TuneReportCheckpointCallback(
{
"binary_error": "eval-binary_error",
"binary_logloss": "eval-binary_logloss",
}
)
],
)
preds = gbm.predict(test_x)
pred_labels = np.rint(preds)
tune.report(
{
"mean_accuracy": sklearn.metrics.accuracy_score(test_y, pred_labels),
"done": True,
}
)
if __name__ == "__main__":
config = {
"objective": "binary",
"metric": ["binary_error", "binary_logloss"],
"verbose": -1,
"boosting_type": tune.grid_search(["gbdt", "dart"]),
"num_leaves": tune.randint(10, 1000),
"learning_rate": tune.loguniform(1e-8, 1e-1),
}
tuner = tune.Tuner(
train_breast_cancer,
tune_config=tune.TuneConfig(
metric="binary_error",
mode="min",
scheduler=ASHAScheduler(),
num_samples=2,
),
param_space=config,
)
results = tuner.fit()
print(f"Best hyperparameters found were: {results.get_best_result().config}")
显示代码单元格输出
Tune 状态
当前时间 | 2025-02-18 17:33:55 |
运行时间 | 00:00:01.27 |
内存 | 25.8/36.0 GiB |
系统信息
使用 AsyncHyperBand: num_stopped=4Bracket: Iter 64.000: -0.1048951048951049 | Iter 16.000: -0.3076923076923077 | Iter 4.000: -0.3076923076923077 | Iter 1.000: -0.32342657342657344
逻辑资源使用:1.0/12 CPU,0/0 GPU
试验状态
试验名称 | 状态 | 位置 | boosting_type | learning_rate | num_leaves | 迭代 | 总时间 (秒) | binary_error | binary_logloss |
---|---|---|---|---|---|---|---|---|---|
train_breast_cancer_945ea_00000 | 已终止 | 127.0.0.1:26189 | gbdt | 0.00372129 | 622 | 100 | 0.0507247 | 0.104895 | 0.45487 |
train_breast_cancer_945ea_00001 | 已终止 | 127.0.0.1:26191 | dart | 0.0065691 | 998 | 1 | 0.013751 | 0.391608 | 0.665636 |
train_breast_cancer_945ea_00002 | 已终止 | 127.0.0.1:26190 | gbdt | 1.17012e-07 | 995 | 1 | 0.0146749 | 0.412587 | 0.68387 |
train_breast_cancer_945ea_00003 | 已终止 | 127.0.0.1:26192 | dart | 0.000194983 | 53 | 1 | 0.00605583 | 0.328671 | 0.6405 |
2025-02-18 17:33:55,300 INFO tune.py:1009 -- Wrote the latest version of all result files and experiment state to '/Users/rdecal/ray_results/train_breast_cancer_2025-02-18_17-33-54' in 0.0035s.
2025-02-18 17:33:55,302 INFO tune.py:1041 -- Total run time: 1.28 seconds (1.27 seconds for the tuning loop).
Best hyperparameters found were: {'objective': 'binary', 'metric': ['binary_error', 'binary_logloss'], 'verbose': -1, 'boosting_type': 'gbdt', 'num_leaves': 622, 'learning_rate': 0.003721286118355498}
这应该给出类似以下输出
Best hyperparameters found were: {'objective': 'binary', 'metric': ['binary_error', 'binary_logloss'], 'verbose': -1, 'boosting_type': 'gbdt', 'num_leaves': 622, 'learning_rate': 0.003721286118355498}